Data annotation is an essential process in the development of artificial intelligence (AI) and machine learning (ML) models. By labeling raw data such as images, text, audio, and video, data annotation allows machines to understand and interpret information more effectively. This process is critical for training models to make accurate predictions and decisions.
As AI continues to evolve, the demand for high-quality annotated data has increased significantly. Businesses across various industries are leveraging data annotation to improve the performance of their AI systems. Whether you're a tech enthusiast, a data scientist, or a business owner, understanding the importance of data annotation and the technologies that support it can help you make informed decisions.
This article provides a detailed review of data annotation technologies, their applications, benefits, and challenges. We'll explore the latest trends in the industry, examine popular tools and platforms, and discuss best practices for implementing data annotation in your projects. By the end of this guide, you'll have a clear understanding of how data annotation can enhance your AI initiatives.
Read also:Understanding The Complexity And Implications Of Fake Doctors Notes
Table of Contents
- What is Data Annotation?
- Types of Data Annotation
- Benefits of Data Annotation
- Challenges in Data Annotation
- Tools for Data Annotation
- Top Data Annotation Tech Reviews
- Best Practices for Data Annotation
- Applications of Data Annotation
- Future of Data Annotation
- Conclusion
What is Data Annotation?
Data annotation refers to the process of adding meaningful labels or tags to raw data to make it understandable for machine learning algorithms. This labeled data serves as the foundation for training AI models, enabling them to recognize patterns and make accurate predictions. The quality of data annotation directly impacts the performance of AI systems, making it a critical component of the development process.
Data annotation can involve various forms of labeling, such as tagging images, transcribing audio, categorizing text, or annotating video frames. The goal is to provide context and meaning to the data, allowing machines to interpret it correctly. This process is particularly important in industries like healthcare, autonomous vehicles, and natural language processing, where precision and accuracy are crucial.
Types of Data Annotation
Image Annotation
Image annotation involves labeling images with specific tags or bounding boxes to identify objects, people, or scenes. This technique is widely used in computer vision applications, such as facial recognition, object detection, and autonomous driving. Some common methods of image annotation include:
- Bounding boxes: Drawing rectangles around objects in an image.
- Polylines and polygons: Outlining complex shapes with multiple points.
- Semantic segmentation: Classifying each pixel in an image into specific categories.
Text Annotation
Text annotation involves labeling text data to identify entities, relationships, or sentiments. This technique is essential for natural language processing (NLP) applications, such as sentiment analysis, named entity recognition, and machine translation. Some common types of text annotation include:
- Part-of-speech tagging: Identifying the grammatical components of text.
- Named entity recognition: Tagging specific entities like names, locations, or organizations.
- Sentiment analysis: Labeling text based on emotional tone or opinions.
Audio Annotation
Audio annotation involves transcribing and labeling audio data to identify speech, sounds, or emotions. This technique is widely used in speech recognition, voice assistants, and emotion detection systems. Some common methods of audio annotation include:
- Speech-to-text transcription: Converting spoken words into written text.
- Speaker diarization: Identifying different speakers in an audio file.
- Emotion detection: Labeling audio based on emotional tone or sentiment.
Benefits of Data Annotation
Data annotation offers numerous advantages for businesses and organizations looking to improve their AI capabilities. Some key benefits include:
Read also:Unveiling The Enchanting Hufflepuff Traits A Deep Dive Into Loyalty Patience And Hard Work
- Improved model accuracy: High-quality annotated data leads to more accurate predictions and decisions.
- Enhanced user experience: Better-performing AI systems provide a more seamless and engaging user experience.
- Increased efficiency: Automated processes powered by AI can streamline operations and reduce costs.
- Competitive advantage: Organizations that leverage data annotation can gain a competitive edge in their industries.
By investing in data annotation, businesses can unlock the full potential of their AI systems and drive innovation in their respective fields.
Challenges in Data Annotation
While data annotation is a powerful tool for enhancing AI models, it also presents several challenges. Some common obstacles include:
- Data quality: Ensuring the accuracy and consistency of annotated data can be time-consuming and costly.
- Scalability: Managing large volumes of data can be difficult, especially for organizations with limited resources.
- Domain expertise: Certain industries, such as healthcare and finance, require specialized knowledge to annotate data effectively.
- Cost: High-quality data annotation services can be expensive, making it challenging for smaller organizations to implement.
Despite these challenges, many organizations are finding ways to overcome them by leveraging advanced tools and platforms, as well as outsourcing to specialized providers.
Tools for Data Annotation
Several tools and platforms are available to assist with data annotation, ranging from open-source solutions to commercial offerings. Some popular tools include:
Labelbox
Labelbox is a comprehensive data annotation platform that supports various types of data, including images, text, and video. It offers advanced features such as collaborative workflows, quality assurance tools, and integration with popular machine learning frameworks.
SuperAnnotate
SuperAnnotate is another powerful platform for data annotation, offering a wide range of features for image and video annotation. It includes automated annotation tools, team collaboration capabilities, and seamless integration with AI workflows.
Amazon SageMaker Ground Truth
Amazon SageMaker Ground Truth is a fully managed service that simplifies the data labeling process. It provides access to a large pool of human annotators and supports automatic data labeling using machine learning models.
Top Data Annotation Tech Reviews
In this section, we'll review some of the top data annotation technologies currently available in the market. These tools have been selected based on their features, ease of use, and overall performance.
1. Labelbox
Labelbox is widely regarded as one of the best data annotation platforms due to its versatility and robust feature set. Its intuitive interface and advanced automation capabilities make it an ideal choice for organizations of all sizes.
2. SuperAnnotate
SuperAnnotate stands out for its focus on image and video annotation, offering cutting-edge tools for object detection, segmentation, and tracking. Its collaborative features and seamless integration with AI workflows make it a top choice for many businesses.
3. Amazon SageMaker Ground Truth
Amazon SageMaker Ground Truth is a strong contender for organizations seeking a scalable and cost-effective solution for data annotation. Its access to a vast network of human annotators and automated labeling capabilities make it a valuable asset for AI development.
Best Practices for Data Annotation
To ensure the success of your data annotation projects, consider following these best practices:
- Define clear objectives: Establish specific goals for your annotation efforts to guide the process.
- Choose the right tools: Select platforms and technologies that align with your needs and budget.
- Ensure data quality: Implement rigorous quality control measures to maintain the accuracy of your annotated data.
- Collaborate effectively: Foster collaboration among team members and stakeholders to streamline workflows and improve efficiency.
By adhering to these best practices, you can maximize the benefits of data annotation and enhance the performance of your AI models.
Applications of Data Annotation
Data annotation has a wide range of applications across various industries. Some notable use cases include:
Healthcare
In healthcare, data annotation is used to label medical images, such as X-rays and MRIs, to assist with diagnosis and treatment planning. It is also employed in natural language processing applications to extract valuable insights from electronic health records.
Autonomous Vehicles
For autonomous vehicles, data annotation plays a critical role in training machine learning models to recognize and respond to various driving scenarios. This includes identifying pedestrians, vehicles, and road signs in real-time.
Retail
In the retail sector, data annotation is used to analyze customer behavior, optimize inventory management, and improve recommendation systems. It helps businesses make data-driven decisions to enhance customer satisfaction and increase sales.
Future of Data Annotation
As AI continues to advance, the role of data annotation in the development of intelligent systems will only grow. Emerging technologies, such as automated data labeling and synthetic data generation, promise to make the process more efficient and cost-effective. Additionally, the increasing demand for specialized annotations in industries like healthcare and finance will drive innovation in the field.
In the coming years, we can expect to see more sophisticated tools and platforms that cater to the unique needs of different industries. These advancements will enable organizations to harness the full potential of AI and drive meaningful impact in their respective fields.
Conclusion
Data annotation is a vital process in the development of artificial intelligence and machine learning models. By labeling raw data, businesses can improve the accuracy and performance of their AI systems, leading to enhanced user experiences and increased efficiency. While challenges such as data quality and scalability exist, the availability of advanced tools and platforms makes it easier than ever to overcome these obstacles.
We encourage readers to explore the tools and technologies discussed in this article and consider how they can be applied to their own projects. To further engage with the topic, we invite you to leave comments, share this article, or explore other resources on our site. Together, we can continue to push the boundaries of AI and unlock new possibilities for the future.
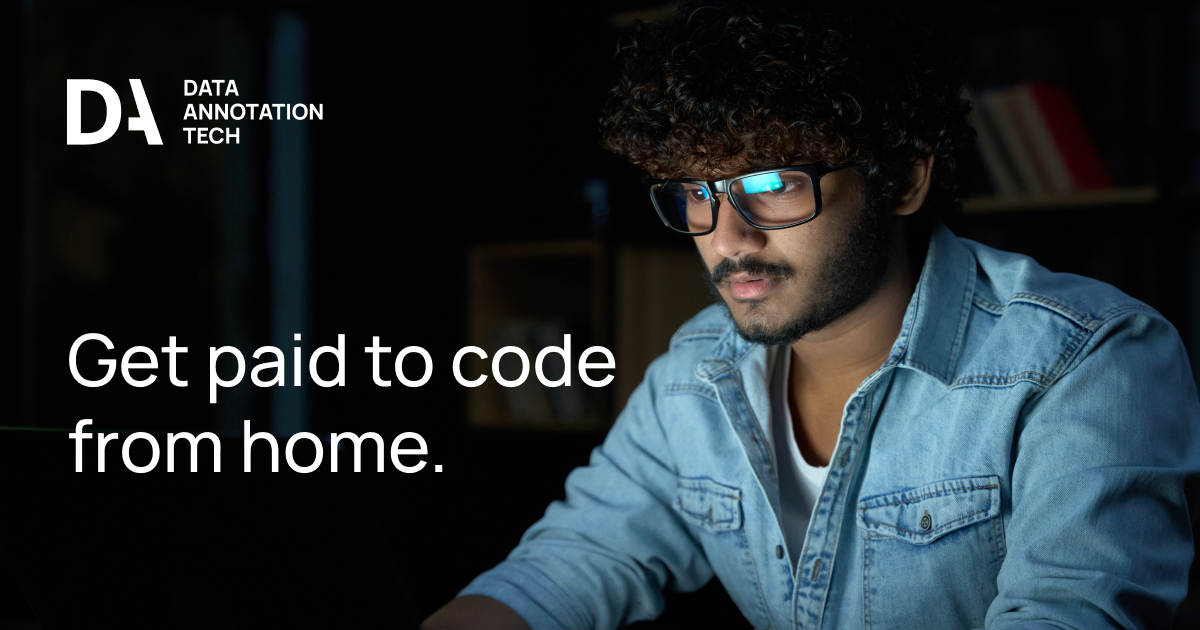
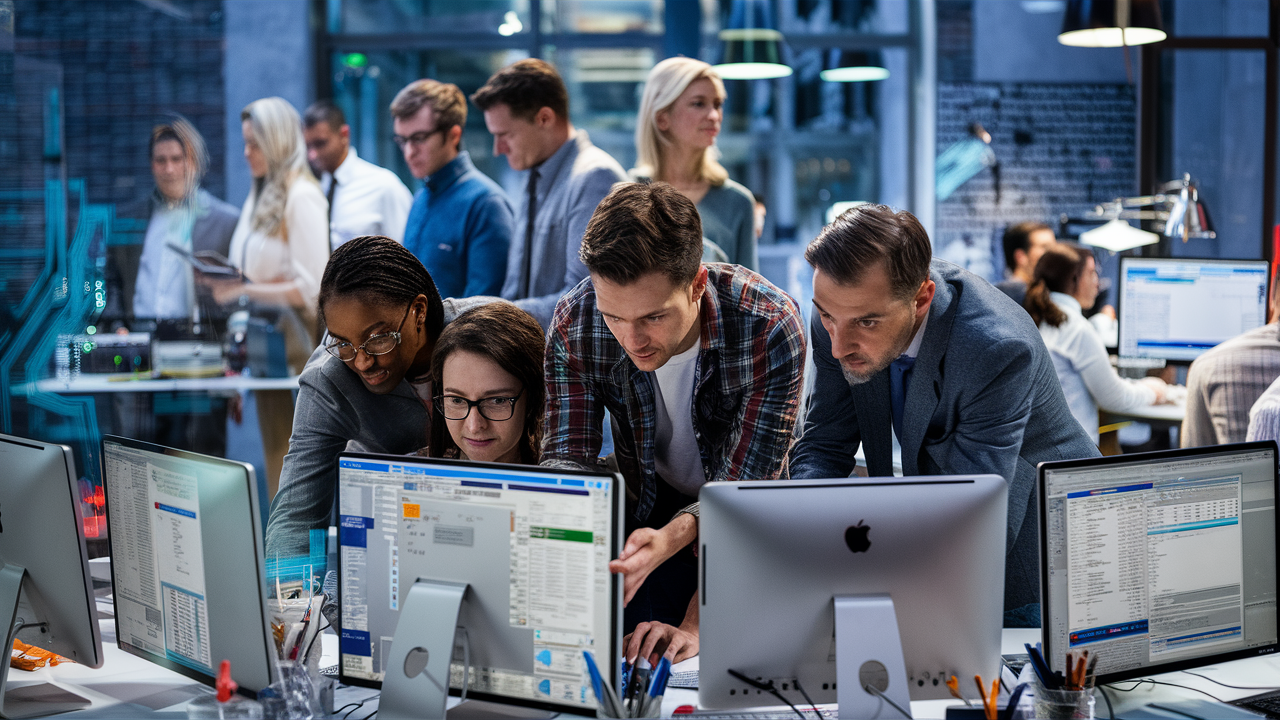
